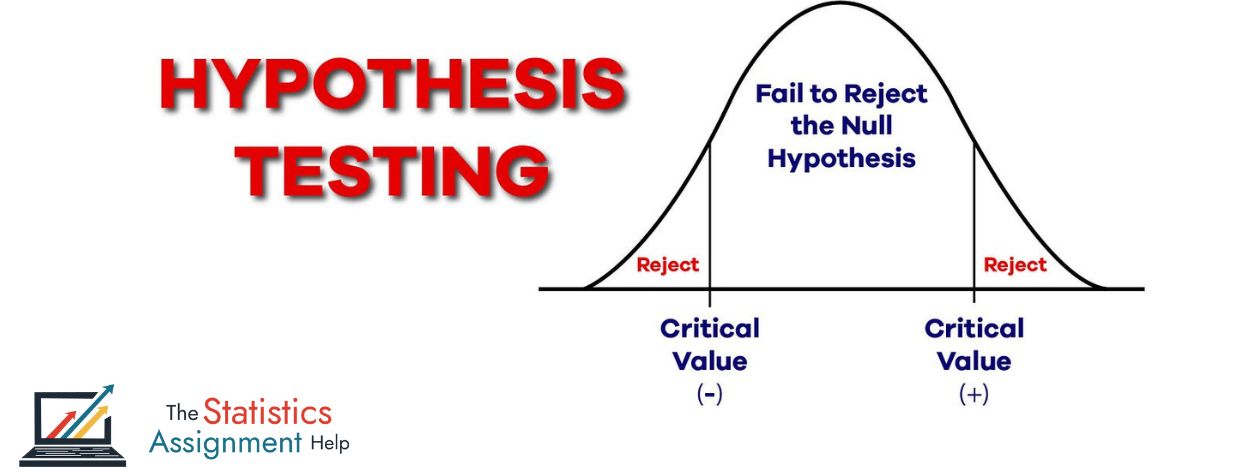
- 5th Mar 2024
- 06:03 am
Within the domain of statistics, hypothesis testing emerges as a potent instrument for informed decision-making and the extraction of meaningful insights from data. Regardless of whether you identify as a researcher, a data scientist, or a student, delving into the nuances of hypothesis testing can markedly improve your capacity to interpret and leverage data proficiently. This in-depth guide aims to explore the core principles and methodologies of hypothesis testing, emphasizing the utilization of statistical software to streamline the entire process.
I. Understanding Hypothesis Testing
Basic Concepts: At its core, hypothesis testing entails drawing conclusions about a population by analyzing a subset of data known as a sample. This analytical process involves the formulation of two primary hypotheses: the null hypothesis (H0), which embodies the default assumption, and the alternative hypothesis (H1), designed to challenge the assertions made by the null hypothesis.
Types of Hypotheses:
- Null Hypothesis (H0): Assumes no effect or no difference.
- Alternative Hypothesis (H1): Posits an effect or a difference.
Levels of Significance:
- Significance levels, denoted by alpha (α), play a pivotal role in hypothesis testing.
- They determine the probability of rejecting the null hypothesis when it is true.
- Common choices for alpha include 0.05 and 0.01.
II. Steps in Hypothesis Testing
Formulating Hypotheses:
- Null Hypothesis (H0): Assumes no effect or difference based on the research question.
- Alternative Hypothesis (H1): Posits an effect or difference, challenging the null hypothesis.
- Consider the nature of the study: Determine whether it is one-tailed or two-tailed.
Choosing the Test Statistic:
- Choose an appropriate test statistic based on the type of data and hypotheses.
- Common tests include:
- t-tests for comparing means or proportions.
- Chi-square tests for analyzing categorical data and independence.
- ANOVA (Analysis of Variance) for comparing means across multiple groups.
Determining the Significance Level:
- Determine the level of significance (α) based on the study's requirements.
- Commonly chosen levels include 0.05 and 0.01, reflecting the threshold for accepting or rejecting the null hypothesis.
Collecting and Analyzing Data:
- Use statistical software to collect and organize relevant data.
- Perform exploratory data analysis to gain insights into the dataset.
Calculating the Test Statistic:
- Apply the chosen test statistic formula to the data.
- Utilize statistical software to automate calculations and minimize errors.
Making a Decision:
- Compare the calculated test statistic with the critical value(s) from the distribution.
- Decide whether to reject or fail to reject the null hypothesis.
III. Common Types of Hypothesis Tests
Z-Tests and T-Tests:
- Z-tests are used for large sample sizes or known population variances.
- T-tests are appropriate for small sample sizes or when population variances are unknown.
Chi-Square Tests:
- Applied to categorical data, assessing the independence or goodness-of-fit.
- Utilize statistical software for efficient chi-square calculations.
ANOVA (Analysis of Variance):
- Compares means of more than two groups simultaneously.
- Statistical software facilitates ANOVA computations and post-hoc tests.
IV. Practical Applications
Medical Research:
- Evaluate the effectiveness of a new treatment compared to a standard.
- Statistical software aids in analyzing clinical trial data.
Market Research:
- Test hypotheses about consumer preferences or market trends.
- Use statistical tools to derive meaningful insights for business decisions.
Quality Control:
- Assess the consistency and reliability of manufacturing processes.
- Employ hypothesis testing to identify and rectify deviations.
Social Sciences:
- Investigate behavioral patterns or societal trends.
- Utilize hypothesis testing to draw conclusions about populations.
V. Challenges and Considerations
Sample Size Determination:
- Adequate sample sizes are crucial for reliable results.
- Statistical software often includes tools for power analysis.
Assumption Checking:
- Validate the assumptions of the chosen test.
- Leverage graphical tools within statistical software for diagnostics.
Interpretation of Results:
- Understand the practical significance of statistical significance.
- Statistical software assists in generating clear visualizations for result interpretation.
VI. Advanced Techniques
Bayesian Hypothesis Testing:
- Embraces Bayesian statistics to update beliefs with new data.
- Statistical software with Bayesian capabilities enhances flexibility.
Machine Learning Integration:
- Explore the synergy between traditional hypothesis testing and machine learning.
- Modern statistical software often integrates both approaches for a comprehensive analysis.
VII. Conclusion
In conclusion, mastering the art of hypothesis testing is essential for extracting meaningful insights from data. Leveraging statistical software not only expedites the process but also enhances accuracy and reliability. As technology continues to advance, the synergy between statistical methodologies and software capabilities opens new avenues for exploration and discovery. Whether you're unraveling the mysteries of medical research, deciphering market trends, or probing societal dynamics, hypothesis testing remains an invaluable ally, and with the right statistical software, the journey becomes not only more efficient but also more enlightening. So, embark on your statistical voyage, armed with knowledge and equipped with cutting-edge tools, and unlock the true potential of hypothesis testing in the world of data analysis.
About The Author:
Name: Dr. Diego Ramirez
Qualification: Dr. Diego Ramirez is a highly qualified expert in statistical analysis, holding a Ph.D. in Statistics.
Academic Background:
Bachelor's Degree: Dr. R. earned his foundational knowledge in statistics through a rigorous Bachelor's Degree program.
Master's Degree: Furthering his academic journey, he pursued a Master's Degree with a focus on advanced statistical methods, solidifying his expertise in the field.
Research Focus:
Dr. Diego Ramirez research focuses on the nuanced aspects of hypothesis testing using statistical software. His work delves into refining and optimizing the application of statistical tests, ensuring robust and reliable results in various research contexts.
Expertise:
With a wealth of experience and a keen understanding of statistical software tools, Dr. Diego Ramirez is recognized for her expertise in conducting hypothesis testing. His proficiency extends to leveraging cutting-edge statistical software for data analysis, contributing valuable insights to the scientific community and beyond.